Researchers in the UK and Sweden have combined density functional theory (DFT) and machine learning to develop a method that accurately predicts the kinetics of nucleophilic aromatic substitution reactions. The new method makes accurate predictions for a reaction class that is challenging to approximate with DFT, but doesn’t require the very large data sets that are typical of pure machine learning methods.
Predicting reaction barriers provides chemists with guidance on reaction kinetics, in both absolute terms like reaction rates and relative terms such as selectivity. However, the nucleophilic aromatic substitution (SNAr) reactions that are common in drug development can proceed by a variety of mechanisms and depend on solvent effects, which methods like DFT can describe poorly. ‘We do a lot of reactivity modelling, and we’re trying to get away from having to model every individual reaction each time, to trying to learn from the data we have,’ comments says David Buttar from AstraZeneca, who led the work. Although machine-learning methods allow efficient, accurate predictions based on existing data, that accuracy depends upon training those methods on data sets, which simply may not exist for a particular research problem.
Buttar and his colleagues have created a hybrid approach. The core of their system is a Gaussian Process Regression (GPR) machine-learning model, which is trained on the known activation barriers of previously-reported SNAr reactions, and learns to predict barriers for unknown but similar reactions. ‘This [GPR model] is more complex than what had been standard in the field,’ notes Heather Kulik, a chemical engineer at the Massachusetts Institute of Technology in the US who studies machine learning in chemistry. ‘It can encode slightly more complex relationships, but not at the upper end of needing to train a full neural network.’
What sets the hybrid approach apart is how much the GPR model gets to know about each reaction. Although the system only takes the reactants, products and conditions as input, it then uses DFT to estimate chemical properties, propose a mechanism, and approximate the energy and characteristics of the transition state. The GPR model receives all of that information about each reaction when it is being trained and making predictions, and those additional insights make the model far more effective.
Buttar and colleagues’ new hybrid system is able to predict reaction barriers with an accuracy of 0.77kcal/mol despite training the model on less than 350 data points. In fact, the full model they developed crosses the chemical accuracy threshold of 1kcal/mol when it’s trained with fewer than 150 data points. A simplified version that doesn’t calculate the properties of the transition states reaches this accuracy with fewer than 200 data points, compared to around 350 data points for a standard machine learning model, which only knows the reactants and products’ chemical structures. These accurate barrier predictions mean the system was able to predict the regioselectivity and chemoselectivity of 87% of reactions it was tested against. ‘If the initial set of descriptors was way off the mark, GPR wouldn’t overcome that hurdle, but in this case it’s a lot of really good information going into the model, and so the model can make predictions on relatively modest data sets,’ says Kulik.
‘The transition state property is a valuable resource for molecular descriptors,’ says Xin Hong, a physical organic chemist at Zhejiang University in China. ‘I believe this hybrid strategy will be a powerful barrier-prediction tool for transformations with rich kinetic data.’ Buttar also notes that the already-trained SNAr model may act as a good starting point to study related reactions or other aspects of SNAr. As does Kulik: ‘They’ve developed a very rich description of key electronic properties that are needed to make the prediction from chemistry over to observed property, and so it makes sense to leverage that mapping for other related properties you might care about in the reaction class.’
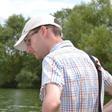
No comments yet