A machine learning algorithm that can predict the mechanical properties of metal–organic frameworks (MOFs) offers a way to overcome these highly varied and versatile materials’ achilles heel – their instability.1 The team behind this work hope that this computational tool will speed up acceptance of these materials by industry.
MOFs are a type of crystalline coordination polymers that form porous structures by combining metal clusters and organic ligands. ‘Their “building block” nature allows chemists to easily tune their syntheses to tailor the pore size and surface chemistry for a specific application,’ explains David Fairén-Jiménez at the University of Cambridge, UK. ‘However, if you wish to use MOFs in real life, you need to shape them into pellets, and this densification may destroy their porosity, thus their functionality.’
‘Although many MOFs are found to be mechanically robust, the reasons behind this are unclear; this systematic study … is important and timely,’ explains Omar Yaghi, a pioneer in MOFs working at the University of California Berkeley, US. Yaghi is also impressed by the way researchers used high-throughput computation and machine learning to explore exactly what makes some MOFs stable.
After training on a dataset of more than 3000 MOFs, the algorithm can accurately predict the mechanical properties of both existing and hypothetical materials. Researchers showed that understanding the different topologies of MOFs is key. ‘In this context, topology studies how the metal clusters are linked with the different organic ligands,’ says Fairén-Jiménez. ‘If we do not take this factor into account, our predictions fail miserably.’ The results back the popular belief among MOF scientists that a higher number of connections in the metallic nodes increases stability, and that certain organic motifs, such as triple bonds, phenyl rings and nitrogen atoms, strengthen the materials.
Fairén-Jiménez has co-founded two start-up companies to exploit MOFs. ‘Last year, we developed monolithic MOFs, a new kind of pellet that, since they are shaped without binders or high pressure, achieve outstanding densities and mechanical properties2,’ he says. ‘Now, this novel computational tool brings us a step closer to start adopting these wonderful porous materials in manufacturing.’
Beyond the algorithm, the team has also created an interactive website – MOF explorer – where chemists ‘can not only visually explore the properties of all the materials we analysed, but also download a little free program that can calculate the properties of newly synthesised MOFs,’ says Fairén-Jiménez. He dreams that, one day, ‘material chemists will have a SciFinder-like tool, where just uploading the crystallographic information will obtain all the properties of the MOF they just prepared’.
References
1 P Z Moghadam et al, Matter, 2019 (DOI: 10.1016/j.matt.2019.03.002 )
2 T Tian et al, Nat. Mater., 2018, 17, 174 (DOI: 10.1038/nmat5050)
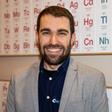
No comments yet