A new robotic platform produces proteins with improved properties in a fully autonomous manner. Moreover, the system is able to experimentally assess these proteins and, thanks to artificial intelligence, interpret the results and then redesign the proteins’ amino acid sequences to boost their functionalities further. In a preliminary test to bolster the thermal tolerance of glycoside hydrolases, the robot increased the proteins’ temperature resistance by at least 12°C.
The platform is designed to improve the properties of proteins in a process that’s ‘identical to the scientific method’, explains project leader Philip Romero, from the University of Wisconsin–Madison in the US. ‘It designs experiments to test hypotheses, sends commands to a fully automated robotic system to execute the experiments, and learns from the resulting data to refine its understanding of the protein,’ says Romero. Eventually, after a few iterations, the system improves the design and the proteins’ performance – in this particular case thermal stability. Overall, this ‘accelerates and automates the process of protein engineering’, notes Romero.
According Jacob Rapp, a PhD student in Romero’s lab who worked on the project, the new system ‘is the first to completely eliminate human involvement in the engineering loop’ and allows a much faster iterative process.
The robots prepare proteins with the exact same molecular machinery as cells, combining and translating a series of previously prepared blocks of DNA. ‘We start with a collection of 34 DNA fragments that can be combined in 1352 different ways, each of which will produce a unique [protein] sequence,’ explains Rapp. In the team’s demonstration, the robot was only provided with information on the experimental thermal stability of six sequences and would select a few possibilities targets to test based on its initial understanding. ‘For each selected sequence, the robot mixes the relevant fragments, stiches them together into a single piece of DNA, … expresses the protein and tests [it] at a range of temperatures,’ Rapp says.
Having made a protein, the system runs an assay to measure thermal stability and then learns from the experiment to optimise its future protein designs. Compared to the six sequences of natural glycoside hydrolases tested, the best sequences selected by the system could withstand at least 12°C. ‘We used glycoside hydrolases as a model, […] now we’re working on applying the same technology to more industrially or clinically relevant proteins,’ says Rapp.
‘I don’t know any other examples for fully autonomous protein design and engineering,’ says Héctor García Martín, an expert in bioengineering based at the Lawrence Berkeley National Laboratory in California, US. ‘It’s a fully automated robotic system that experimentally tests the designed proteins and uses that information to drive the next engineering cycle,’ he adds.
Rapp draws comparisons with the Nobel prize-winning protein engineering technique directed evolution. He points out that while directed evolution relies on a series of small steps towards a top performing protein, usually making a few mutations in each round, the new robotic system averages over 100 mutations in each iteration. Additionally, because the autonomous synthetic system operates on a cell-free approach, it could create ‘killer’ proteins – ones that would compromise the viability of a biological cell but that could still have possible applications in the laboratory.
However, the two techniques are not necessarily competitors. ‘[Researchers could] explore an extremely exciting paradigm – integrating machine learning with directed evolution,’ says Marc Güell, an expert in synthetic biology at Pompeu Fabra University in Barcelona, Spain. For Güell, the application of artificial intelligence to protein design is the biggest tipping point in synthetic biology since Crispr. Having an automated system ‘with the capacity to “speak” protein’ is very powerful, he says. ‘The possibility to influence evolutionary trajectories with artificial intelligence could be transformational, [such] operational optimisation could increase the speed of protein engineering,’ adds Güell. ‘It takes automation one step further.’
References
J T Rapp et al, Nat. Chem. Eng. 2024, 1, 97, DOI: 10.1038/s44286-023-00002-4
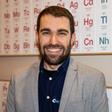
No comments yet