New computational method learns how to estimate bond energies as well as a trained synthetic chemist
‘Chemical bonds are only defined by the minds of good chemists,’ says John Parkhill, researcher at the University of Notre Dame in Indiana, US. But his latest study turns this idea on its head. Parkhill’s team has designed a neural network – a sophisticated computer program that mimics the behaviour of a real-life brain – which is able to accurately determine bond energies.1
Parkhill and his team created a piece of software that breaks down molecules, then analyses and classifies single bonds according to their different electronic properties. Next, chemists taught the neural network using an existing database of over 130,000 molecules.2 With this information, and combining it with a series of different algorithms, the programme is able to predict bond energies, often beating the intuition of trained synthetic chemists.
‘Neural networks can be used to make quantitative models of chemical concepts that are not possible with just quantum mechanics,’ explains Parkhill. ‘[Before], I got asked all the time: “How much stronger is this bond?”. […] Now I can answer that question, because my machine learnt chemical bonding concepts.’
According to Parkhill, neural networks can compete with classic ab initio models because they are cheaper but still retain accuracy. Moreover, ‘neural networks can make quantitative models of abstract concepts,’ Parkhill comments. ‘Chemistry is full of useful but nebulous concepts that defy precise physical definition, most famously chemical bonds.’ Soon, the advances in neural networks will allow chemists to answer questions that ‘cannot be answered with physics alone’.
Alan Aspuru-Guzik, a theoretical chemist at Harvard University, US, believes ‘the paper by Parkhill is very exciting from several angles’. He explains how ‘their representation can be readily extended and combined with other approaches […] to generate novel molecular structures in design applications – something that interests many of us in the community’. The accuracy of this new method amazes Aspuru-Guzik. ‘Chemical accuracy is the gold standard of quantum chemistry. [What] Parkhill and co-workers reached is fabulous.’
Neural networks are useful tools, even comparable to human intuition, but Parkhill and Aspuru-Guzik agree they cannot replace trained chemists. ‘Chemists [can] make rational choices about molecular structures in the same way a chess grandmaster makes decisions about chess. An artificial intelligence system may predict better energies or geometries, but may not be able to make decisions,’ says Aspuru-Guzik. ‘[Our network] predicts [data] quantitatively and reproducibly. It saves chemists from the impossible tedium of predicting an energy a billion times,’ explains Parkhill. ‘And [it] never messes up because it has the flu,’ he jokes.
Neural networks could have many applications in the design of new drugs and materials – Aspuru-Guzik and his team used them last year to create novel organic light emitting diodes.3 Parkhill also thinks these tools could enter the classroom. ‘[Neural networks] might be useful in organic chemistry classes,’ he says. ‘Even I learnt something I should have known – the single bond between alkynes is one of the strongest single bonds.’
References
1 K Yao et al, J. Phys. Chem. Lett., 2017, 8, 2689 (DOI: 10.1021/acs.jpclett.7b01072)
2 R Ramakrishnan et al, Sci. Data, 2014, 1, 140022 (DOI: 10.1038/sdata.2014.22)
3 R Gómez-Bombarelli et al, Nat. Mater., 2016, 15, 1120 (DOI: 10.1038/nmat4717)
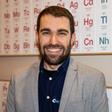
No comments yet