It’s almost 20 years since mathematician Clive Humby coined the analogy ‘data is the new oil’. The original idea was that oil powered the last industrial revolution, and data seems set to drive the next one. It’s a catchy slogan that has taken hold in recent years and is partly responsible for transforming perceptions of data from worthless by-product to a vital commodity that will fuel advances in everything from research to business and government.
The analogy also works because making something useful out of data or oil involves a complex process – getting hold of them is just the first of many steps. But if we take a closer look at each of those steps, there are also important differences that reveal potential pitfalls and misconceptions about how data is viewed and used.
If you treat data just like oil, you’re going to miss the opportunity to really drive innovation.
Prospecting
Oil is a finite resource and finding it is increasingly difficult. As long as our economy relies on the products of oil exploration the price per barrel will trend upwards. By contrast, finding large volumes of data is easy. In chemicals manufacturing, more sensors are collecting more data on more things, more often. But the useful information content is not increasing at anything like the same rate. The challenge with data prospecting is to find the data to solve the problem at hand.
Sometimes no amount of existing data will help. Then we have to generate the data using expensive experiments. However, this can still be economically viable because tiny amounts of quality data can have huge value. A few rows and columns of data from a well-designed experiment might yield the optimised recipe for a low-cost fermentation growth media, which is the key to commercialising a lucrative new biotech product.
The big difference
Oil is scarce but it’s always valuable, data is abundant but only some of it is valuable
Extraction
Once a well has been drilled, most of the oil finds its way out of the ground by itself. Similarly, it’s fairly simple to maintain a regular flow from an established data well. The difference in many use cases is that we will need to tap multiple sources of data and combine them, which requires complex pipework with different connectors.
Establishing and running an oil well is incredibly expensive. The proposed Rosebank oil field, west of Shetland, is projected to cost a staggering $9.8 billion over its life. However, the expected return is 300 million barrels, worth $30 billion at $100 per barrel. While these are only estimates, the risks are well enough understood to justify the investment. The risk analysis for data extraction is completely different because the cost is comparatively tiny but the returns are also smaller and much more uncertain.
The expectation that any data is valuable leads to bad decisions about where to invest
That means careful planning is needed beforehand to extract or generate data with useful information content. First by defining the question that we need to answer, and then designing a model to answer the question. Only then should we think about what data is required to build the model.
For example, a company developing catalysts to turn carbon dioxide into polymers needed to improve cost efficiency to make the process commercially viable. They understood that they needed a model relating the recipe factors to the yield and impurity levels in the product. Knowing this they were able to identify relevant existing data and design experiments to deliver the missing data efficiently. That model quickly found a solution that maximised yield and minimised the costly by-products.
The big difference
Oil wells extract as much oil as is profitable; extracting every bit of data is wasteful so careful planning is needed
Processing and refinement
Data and oil both need to be cleaned, refined and processed to turn them into useful products. Data processing will often include summarising to distil out the most useful fractions or transformation to synthesise something with more useful features and properties.
Yet for oil these activities will be much the same at any refinery. With data there is an ever-growing diversity of end products and so little standardisation of the process. Analytics-ready data will often have been through multiple cycles of end-use testing and refinement of the source data that is extracted. This tight coupling between these stages means that people and teams need the tools and skills to rapidly iterate through extraction, refinement and end-use.
The big difference
Oil processing is universal and standardised; but the diversity of end products for data means refinement is rarely standardised
Products and end-use
There is a diversity of products derived from crude oil, although the vast majority is still burned as fuel producing harmful pollutants. Their markets are mature, and the value of those products is relatively fixed and predictable.
The end products of data are just as diverse. In the chemical industries alone, data can accelerate technologies to market, ensure consistent quality, minimise costs and maximise efficiency. But it is not always easy to put a price on these outcomes.
We should recognise that a lot of data is less like oil and more like soot – an amorphous, inert waste product
Poor statistical and data literacy within organisations presents additional problems. While most people can grasp how drilling for oil ultimately enables planes to fly, the process of generating insights from raw data is like magic to many. This leads to the expectation that any data is valuable, which leads to bad decisions about where to invest time and money. A gusher of data from sensors on a manufacturing process might seem like an unexploited resource but if they only report that the process is operating within set parameters, there will be no value in further exploration.
Another big difference is that data products can be re-used: a model created to develop a chemical manufacturing process can later be used to meet a demand for a product with new target properties. These products can be augmented and improved as new data is made available.
The big difference
Oil products have established value chains but poor circularity; data value chains can be less well established but the products have good circularity
Chemists should be jewel hunters, not oil barons
We should recognise that a lot of data is less like oil and more like soot – an amorphous, inert waste product that can’t economically be converted into anything useful.
At the same time we need to be able to recognise priceless gems when we see them. Chemists need to appreciate the clarity that comes from a few carats of flawlessly structured data. They need to be able to find the diamonds in the soot.
You can become a better data explorer by investing in Statistical Thinking for Industrial Problem Solving with this free online course from JMP. And see how scientists and leaders at Johnson Matthey are advancing their digital transformation with data-driven science and Design of Experiments in this interview with Chemistry World.
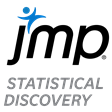
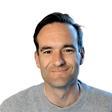
No comments yet