There is justified excitement about how AI can speed the pace of innovation in chemical and materials science. One indicator of this is the effort and funding being put into the development of fully autonomous or ‘self-driving’ labs. Take the A-Lab, for example – an autonomous laboratory developed at Lawrence Berkeley National Laboratory in the US, that recently synthesised a raft of novel materials.
The A-Lab study is an impressive achievement, but it has also generated a lot of discussion about the pros and cons of automated R&D. That’s because while these approaches offer productivity gains and better quality results, they also pose some important questions. How much of the scientific process should be automated, for example, and do we want to take people out of the loop entirely?
I think we’ve only scratched the surface of our potential with human intelligence. So we should focus on working out how AI and lab automation can best augment our existing tool set and empower better human-driven innovation.
In the loop
Closed-loop optimisation is a big part of some people’s vision of a lab of the future. The idea is that experiments are run and analysed by automated hardware, and the data is interpreted by algorithms that decide the next recipe to try. There is no human intervention at any point.
These self-driving labs have obvious advantages. They can work all day, every day and don’t require lighting or large, heated lab spaces. And they can work smarter: algorithms are highly efficient at exploring possibilities over many factors in high-dimensional spaces that the human mind struggles to grasp. In the A-lab example, 41 new compounds were synthesised in just over two weeks, which the authors say is 50–100 times more efficient than a human.
It’s naïve to assume that we can automate away the work we don’t enjoy and keep the bits we like
They also appear to offer chemists relief from tiresome or repetitive manual tasks. In my days in the lab I would have gladly handed over much of the work to a robot: making up dispersions to optimise pigment milling was incredibly messy – my lab coat looked like a Jackson Pollock painting – and repeatedly taking and analysing samples was tedious. Freed of that drudgery, I imagined I could then spend my time poring over the results and deciding what to try next. Yet it’s naïve to assume that we can just automate away the work we don’t enjoy and keep the bits we like – that’s not how disruption works. Instead, we should think critically about where machines and humans can add the most value.
Only human
There are some jobs where humans have better skills, such as deciding on the best compromise when there are multiple objectives. This is often a business decision, requiring feedback from different stakeholders as priorities and opportunities evolve. Similarly, determining the project end-point is hard for an algorithm. Deciding when you have sufficient confidence in a solution, or at what point to terminate a project to cut losses and redeploy resource are choices humans should make.
Closed-loop systems can be biased towards chemistry that is easily automated
AI could suggest useful reaction conditions or formulations where there is relevant data or literature. But humans can also bring less codifiable knowledge from theory, experience and even intuition – biases are not always bad. And if the data suggests better outcomes by exploring higher temperatures, for example, then a human should make the choice on how to proceed safely. Ironically, closed-loop experimentation can also be limiting because it is biased towards chemistry that can be easily automated, like looking for your lost keys under a lamppost because the light is best there.
The critical discussion surrounding the A-Lab study also highlights other limitations. The characterisation work used to confirm the syntheses was criticised for being low quality. As one of the authors acknowledged in their response, humans would certainly have done a better job of that work. But they also emphasised that ‘the A-Lab is not intended to replace the materials discovery process with AI agents … A human in the loop is still required.’ A hybrid solution is therefore needed that leverages the best qualities of machines to lower the barriers for humans to exploit data-driven science.
I no longer work at the bench. But the area where I do work is also going to benefit from humans and machines collaborating. Statistical Design and Analysis of Experiments is an established method for accelerating innovation that is underutilised, partly because scientists find the concepts difficult and the terminology impenetrable. It is not hard to imagine how large language models could help a scientist to translate their goals into a statistically optimal experimental plan with machine instructions to automate the lab work. This still requires curious scientists that are comfortable with how you can learn from data using statistical modelling and machine learning and so able to form the most useful working partnership with the machines.
Disruption from AI and automation is inevitable but we can’t know exactly what this will mean for lab science. Improving data literacy within the chemical sciences will be important preparation for an uncertain future and help to ensure that humans stay at the heart of scientific progress. JMP’s webinar series on data and Design of Experiments is a great place to begin. We can start preparing today for whatever tomorrow will bring.
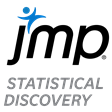
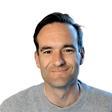
No comments yet