Software synthesis suggestions are hampered by biased and incomplete datasets
I have a confession to make: several years ago, I was rather optimistic about retrosynthesis software for organic chemists. That enthusiasm has cooled a bit. For those in the crowd who aren’t synthetic organic chemists, ‘retrosynthesis’ refers to the working-backwards mode of thought that you need to use when planning out how to make a given molecule. Looking at the desired final structure, you think about what some plausible earlier steps in its synthesis might be. Perhaps that six-membered ring could be formed using a cycloaddition reaction, or that amine might get installed from what used to be an aldehyde? You can probably come up with several tentative lines leading backwards to more simple starting materials, and it’s up to you to work out which of these are the most promising.
The idea, though, was that machine-learning based software could make those decisions more its problems than yours. There is, of course, a huge corpus of literature in this field – a century or more of reactions, reagents, conditions, and transformations – and it seems like just the thing to have software internalise all this information and put it to use. Organic chemists could use the help, to be honest: the literature has long grown past the ability of anyone to grasp it all (see Chemistry World, August 2023, p21), and new reactions just keep coming. A program that could tirelessly search through this mountain of data would be a wonderful thing to watch in action.
Even when failed experiments are available, there’s no guarantee that they failed because the underlying reaction wasn’t sound
There are several commercial packages that are made to do just that. So why do I have misgivings? If you’d asked me five or six years ago, when these started to come to market, I honestly would have hoped for more progress by now than we’re actually seeing. The programs are improving, and they do have their uses, but I can’t help but think that they’re not fulfilling their potential yet.
And there are some real reasons for that, rooted in the depths of the gigantic literature pile itself. One of these is the negative data problem (see Chemistry World, March 2023, p25). Machine-learning algorithms can’t get traction if you only feed them things that worked; they need to see what didn’t work as well. And we chemists tend not to publish so many of those, unfortunately. There’s a built-in bias for talking about the successful parts of a project rather than exhaustively listing all the failures, but an exhaustive list of the failures is exactly what the software needs in its diet. And there’s a more subtle problem. Even when failed experiments are available, there’s no guarantee that they failed because the underlying reaction wasn’t sound. There are a lot of ways for reactions not to work – contamination, for example, ranging from adventitious water or oxygen all the way up to bad batches of reagents or solvents. This confuses the algorithms, as you could well imagine. As do the papers that are just flat-out wrong, and there are more than a few of those out there.
We may have to go back and recapitulate more of the literature under controlled automated conditions
That’s not all. A paper last year from some retrosynthesis software pioneers pointed out that the organic chemistry literature is shot through with unintended biases. A machine learning algorithm might see a particular reagent showing up in a whole list of different reactions from different groups and conclude that it’s highly effective – but it might well be just picking up the results of popularity or easy availability instead. The unspoken assumption that machine learning makes is that the reactions it’s building on were the result of sustained thought and effort, when they might be the result of what happened to be on the shelf. Or what wasn’t back-ordered. Or what was cheap, or what those folks down the hall used that time a while back. Since the literature was assembled by humans, it is soaking in human frailties. As the philosopher Immanuel Kant put it, ‘Out of the crooked timber of humanity no straight thing was ever made’.
What to do, then? We may have to go back and recapitulate more of the literature under controlled automated conditions in order to build something that we can actually trust. The positive data will be reliable, and the negative data won’t be discarded. One set of machines will produce the perfect material to feed to the other set, while we human chemists cheer them on.
References
W Beker et al, J. Am. Chem. Soc., 2022, 144, 4819 (DOI: 10.1021/jacs.1c12005)
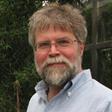
No comments yet