Our tools for understanding living systems have advanced hugely in my lifetime. In just the last two decades the cost of sequencing a whole human genome has gone from one hundred million USD to less than a thousand and in turn the volumes of data being generated have increased enormously. Chemical innovations like sequencing techniques, fluorescent tags and biorthogonal reactions have been pivotal to many of these advances and collaboration between the disciplines is sure to bring more. Yet chemistry could also benefit from borrowing some ideas from biology.
Specifically, I think you would have to concede that biologists are ahead of chemists in the race to digitalise empirical learning. Never mind the ‘lab of the future’, there are commercial biology labs right now in which automation of large and complex biological experiments is fairly routine. While the benefits that they are realising can provide a useful direction as chemistry is more gradually digitalised, we can also learn from some of the less effective uses of these innovations.
Biology has been quick to realise the benefits here, in part because of the nature of its research. Biologists deal with complex, interconnected systems and emergent properties, so they need big experiments to explore and deconvolute that complexity to understand the many factors that might be involved. For example, if you need to understand how point mutations affect the activity of a protein, you might well find that the effect of changing amino acid at one position will depend on what amino acids are present at other positions. Exploring that possibility space can be done more efficiently and with much less drudgery by using automation and high-throughput approaches that are ideally suited to large-scale, repetitive protocols.
Digital innovations are bringing orders of magnitude improvements in the quality, volume, and rate of collection of data
Biology experiments also have some inherent advantages here: they tend to involve a fairly narrow range of operations, the solvent is always water, and heating is usually limited to not much warmer than room temperature. The enabling technologies that have made the most impact are therefore largely about accurately dispensing small amounts of different watery ingredients into very small vessels. And fluorescent probes have become so important precisely because they enable the outcomes of huge numbers of experiments to be tracked simultaneously using fairly simple imaging technology.
Digital tools also overcome one of biology’s big challenges. Living systems are noisy, which can lead to both false positives and false negatives, so “Do everything in triplicate” is the standard in biology. Anything that reduces random or systematic error and boosts the signal to noise ratio is very welcome. Today’s lab robots are highly valued for their ability to consistently repeat simple but critical tasks like pipetting.
The other big benefit seen in digital biology is not strictly about automation. Digitalised experiments enforce the capture of the instructions in a way that can be easily structured to maximise learning. When all the relevant lab operations are explicitly coded in the experimental plan they can easily be turned into features for data-driven models. And no matter who runs the experiment, the instructions will be the same, so the results are more reliable. The instructions can also be shared with other scientists, making the science more reproducible. It’s even more powerful when you can also automate the capture of the outcomes and the data flows from different hardware.
The capability to simultaneously test hundreds or even thousands of different possibilities means biologists can now ask questions that were previously unanswerable. Surely chemists want this too? Digitalising the execution of the full diversity of chemistry experiments is going to be much more challenging, but we can still learn a lot from biology – including how to avoid the pitfalls.
We should always focus on getting the most from our experiments and making sure that every run counts
Digitalised experimentation is a new paradigm and we should expect some missteps as we adapt our methods and mindset. As a development chemist I used to spend three days on a single trial, so the latest capabilities are mind-blowing to me, and I am not surprised that people get over-excited by the promise of massively increased experimental throughput. Yet doing more experiments and capturing more data does not automatically lead to better science. In fact, these approaches can end up being more wasteful if a project isn’t designed to make the most of their potential.
Statistical design and analysis of experiments or DOE has proven to be a valuable method in chemistry since the 1950s. It ensures that you maximise your learning, especially in situations where it is only feasible to test a handful of all the possible combinations of factor settings because doing so is laborious, time-consuming and expensive. DOE will continue to be important because these practical constraints are still the norm for most lab work in chemistry R&D and it will take time for automation to change that. And sticking with a DOE mindset will ensure efficiency as we move towards fully digital chemistry experiments.
Digital innovations are bringing about orders of magnitude improvements in the quality, volume, and rate of collection of data for empirical learning in biology. It will be very exciting and challenging to adapt as we start to see the same in chemistry. But we should always focus on getting the most from our experiments and making sure that every run counts.
If you are not already using DOE, you can get an introduction to this valuable tool in a free online workshop featuring statistical experts from JMP. Find out more and register in the Chemistry World Design of Experiments collection in partnership with JMP.
Phil Kay, Learning manager at JMP
Phil loves showing people how data analytics enables better science. His passion for this started with some big successes using statistical design of experiments as a development chemist at FujiFilm. Phil has a master’s degree in applied statistics and a master’s and PhD in chemistry. He is a chartered chemist and chair of the RSC process chemistry and technology interest group.
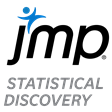
No comments yet