A critique of a paper published in Nature last year, which reported the discovery of over 40 novel materials using an autonomous laboratory guided by artificial intelligence, has concluded that no new materials were discovered.
The laboratory, dubbed A-Lab, was developed by a team led by Yan Zeng and Gerbrand Ceder at Lawrence Berkeley National Laboratory, US, in collaboration with researchers from Google DeepMind, and used machine learning tools to plan and interpret the outcomes of experiments performed by robots.
The team reported that, over 17 days of independent operation, A-Lab performed 21 experiments a day and produced 41 new inorganic compounds out of an attempted 58 – a success rate of 71%. The researchers said that this success rate could be improved further with minor modifications to the lab’s computational techniques and its decision-making algorithm.
However, soon after the A-Lab paper was published at the end of November 2023, Robert Palgrave, a materials chemist at University College London, UK, highlighted ‘very serious problems’ with it, expressing concerns over the quality of the experimental analysis.
After initially sharing his concerns on X, formerly known as Twitter, Palgrave was contacted by Leslie Schoop, a materials chemist at Princeton University in the US, who asked if he wanted to work together on a more complete analysis of the A-Lab paper. That analysis has now been posted as a preprint on the ChemRxiv server.
Having attempted to repeat the original analysis carried out by A-Lab, Palgrave and Schoop now report finding ‘systematic errors all the way through’ which, Palgrave says, could be put down to issues with both the computational and experimental work.
‘They couldn’t deal with the issue of compositional disorder,’ explains Palgrave. ‘This is where you have a crystal structure where you have the atoms arranged in an ordered pattern, but within that pattern there can still be disorder.’
‘Compositional disorder happens in a massive amount of compounds in solid state chemistry … but it’s difficult to deal with computationally,’ he adds. ‘Computationally, it’s much easier to calculate completely ordered things … when you add this layer of disorder, it makes the calculations much harder.’
Palgrave and his colleagues found that none of the structures the A-Lab team had calculated had compositional disorder. ‘What they didn’t realise is that two thirds of their entire predictions were just ordered versions of compounds that were already known to be disordered.’
Palgrave also highlights issues with the x-ray diffraction (XRD) analysis in the original report, in particular due to the use of AI to carry out Rietveld refinement; a technique used in the characterisation of crystalline materials. Palgrave and Schoop conclude that the AI was not able to conduct the task well enough to accurately identify the compounds.
‘[The Rietveld refinement] was very bad, very beginner, completely novice human level – that led to them misidentifying things in some cases and not understanding what compounds were in their mixture,’ says Palgrave. ‘So, ultimately, unfortunately, it’s likely they didn’t make any discoveries.’
‘In the last 12 months, AI has made big advances and I think it’s going to have a big impact in probably every field, including solid-state chemistry,’ he adds. ‘But there is a bit of a tendency to feel that AI has to change everything right now … and probably the message is that it’s going to take longer in more technical fields and we shouldn’t expect everything to change overnight.’
Because the AI didn’t recognise that substitution and site mixing can occur, it assumed that because the composition was different, these were “new compounds”
Susan Latturner, Florida State University
Susan Latturner, an expert in solid-state chemistry at Florida State University, in the US, says the approach laid out in the A-Lab paper was ‘very interesting’ and ‘worthy of high-level publication’. However, she adds that under current conditions the approach seems to be more effective with molecular compounds, rather than inorganic solids.
‘This is due to the fact that when the composition of a molecular compound is varied, its molecular packing, crystal structure and properties will change completely in a way that is very obvious,’ she explains. ‘But if you are doing a similar thing with an extended inorganic solid, you can get low level random substitution or doping – the overall structure can stay the same, it looks like the same material, but the properties can change dramatically.’
‘This is what Palgrave noticed when looking at the powder x-ray diffraction patterns. Many of the PXRD patterns of different products made by the A-Lab were identical. The A-Lab had made several substituted variants of the same compound, which maintained the same structure,’ adds Latturner. ‘But because the AI didn’t recognise that substitution and site mixing can occur, it assumed that because the composition was different, these were “new compounds”.’
Latturner concludes that, in order to properly analyse diffraction patterns, AI systems will need to be trained to handle the possibility of site mixing. ‘Until then, a knowledgeable human is needed to analyse the data, compare to databases and literature, and determine what was actually made,’ she says.
Chemistry World approached the Ceder lab for comment but did not receive a response in time for publication.
In December 2023, Ceder posted a response to Palgrave’s original X analysis on LinkedIn. In this post he provided additional data that he argues confirms that A-Lab had successfully synthesised the compounds reported in the paper. However, Ceder also highlighted that it was never the team’s intention to replace the materials discovery process with AI agents.
‘We have no doubt that a human can perform a higher-quality refinement on these samples,’ he wrote. ‘However, it was our objective to show what an autonomous laboratory can achieve, not to demonstrate what the best (or average) human can do externally to the A-Lab.’
References
J Leeman et al, ChemRxiv, 2024, DOI: 10.26434/chemrxiv-2024-5p9j4
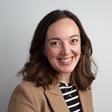
No comments yet