Atomic scale simulations are making it possible to do more with complex chemistry than ever before. Such technology will be a vital component of the sustainable future, both boosting efficiency for chemists at an individual level, and allowing companies to handle increasingly large amounts of data while harnessing the numerous benefits of AI.
During this webinar, we’ll reveal how machine learning-based atomic scale simulations performed with Matlantis help rationalise experimental results, focusing on CO₂ storage.
The hour-long event will begin with an introduction to Matlantis by Dr Rudy Coquet from PFCC – the start-up behind Matlantis. Professor Masaki Kawano from Tokyo Tech will then explain how a copper-based coordination network selectively adsorbed CO₂ at room temperature and retained it for over a week while exposed to the atmosphere.
The case study will shed some intriguing insights, such as how the CO₂ adsorption energy and diffusion mechanism throughout the network were simulated using Matlantis, which helped to rationalise the experimental results. For example, it was found that CO₂ enters isolated voids through transient channels termed ‘magic doors’. In situ powder X-ray diffraction revealed no crystal structure change before and after CO₂ capture.
This webinar will be especially suitable for computational scientists who face the limitations of current simulation approaches and anyone interested in game-changing atomic scale simulations. No prior knowledge of the subject is required, and at the end of the webinar your questions will be put to our speakers by the host.
During this hour-long interactive webinar you will:
- Discover how Cu-based coordination networks with isolated pores but no interconnecting channels selectively adsorb CO₂ under ambient conditions
- Learn how machine learning-based atomic scale simulations with Matlantis help rationalise experimental results
- Take your first step towards large-scale materials discovery, supported by state-of-the-art neural network potentials
Masaki Kawano
Rudy Coquet
PFCC’s mission is to contribute to a sustainable future by enabling companies to create innovative materials. To achieve this, they have combined Preferred Networks’ artificial intelligence expertise and computing infrastructure with ENEOS’s expertise in chemistry to build their product. While computational chemistry has recently made substantial achievements, some bottlenecks persisted: the long computing time, problem-specific methodologies, and high-performance computing environment. Matlantis solves these problems with the fast, versatile calculation engine and browser-based user interface with no need for a special environment. They believe Matlantis will not only elucidate experiment results but help researchers discover and invent new materials, and sheds light on unknown principles.
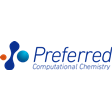