An active learning algorithm has discovered high-entropy versions of Invar alloys – materials widely used for scientific instruments and industrial transportation of liquefied gases because of their tiny thermal expansion. The technique could have significant potential for searching huge ranges of potential material compositions to find a small number with desirable properties.
The 1895 discovery of Invar (from invariant) – an iron–nickel alloy whose thermal expansion coefficient drops suddenly at a nickel composition of about 30–45% – earned Charles-Edouard Guillaume of the International Bureau of Weights and Measures the 1920 Nobel prize for physics. However, although it has exceptional thermal stability, its mechanical properties such as strength and ductility are less impressive. Alternative ‘Invar alloys’ are often prohibitively expensive or have other disadvantages.
Researchers have recently begun to seek candidates among the high-entropy alloys – unconventional mixtures containing multiple metals in significant proportions rather than a single principal element. ‘Previously people thought that when you mixed more elements in an alloy you’d get many precipitates, these precipitates would make your alloy super-brittle and it couldn’t be used for any application,’ explains materials scientist Ziyuan Rao of the Max-Planck Institute for Iron Research in Düsseldorf, Germany. ‘But in 2004 scientists found that when you have certain special element combinations you can get a solid solution – it was a very surprising result.’ The problem is that the composition space of possible high-entropy alloys is effectively infinite, so searching by hand for the combination that yields the optimum value of a specific property is near-impossible.
Rao and colleagues led by Dierk Raabe therefore used a self-optimising machine learning algorithm. These artificial neural networks, which make predictions based on an initial experimental dataset, compare their predictions against more experimental data and effectively teach themselves to refine their predictions, are becoming widely used in chemistry and other fields. However, the initial experimental dataset was insufficient to train the algorithm. The researchers therefore used an ‘active learning’ approach. ‘We predict not just the thermal expansion coefficient: we also predict the uncertainty,’ explains Rao. ‘We did experiments with alloys with very large uncertainty, and by this we could improve the performance of our model.’
From their initial dataset, their algorithm picked out 1000 potentially promising – but highly uncertain – candidates for more sophisticated computational screening such as density functional theory. Those that looked most interesting were then cast before the results were added to the initial dataset. Eventually the researchers found two high-entropy Invar alloys of iron, nickel, cobalt and chromium with room temperature thermal expansion coefficients comparable to those of Fe65Ni35 – the optimum composition for Invar. The researchers are now studying the other properties of their material. ‘Chromium, for example, is often used to improve the corrosion behaviour of stainless steel, so it may have better corrosion behaviour than traditional Invar alloys,’ says Rao.
Computational materials scientist Prasanna Balachandran of the University of Virginia in the US says that ‘the concept of using uncertainties to guide new materials discoveries is not new’, but ‘the sheer vastness of this search space and the fact that they could navigate this space and find potentially interesting materials with better properties is the remarkable thing’. He would now like to learn more about what the machine was learning in each subsequent step, pointing out that the theoretically predicted thermal expansion coefficients do not agree very well with the experimentally measured ones. ‘We have seen similar results in the past,’ he says. ‘The fact that you don’t need to have an accurate model to discover new and unexpected materials with better properties is a mystery.’
References
Z Rao et al, Science, 2022, 378, 78 (DOI: 10.1126/science.abo4940)
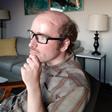
No comments yet