It’s been a year since ChatGPT burst onto the world stage. The AI-powered text generator was notable for its convincing and natural-sounding responses and it quickly proved popular – it’s now approaching 200 million users. But after a year the question remains whether the large language models (LLMs) that enable tools such as ChatGPT will prove useful to science or are simply a distraction.
‘Chatbots’ aren’t a new innovation and can be traced back as far as the 1960s. However, the ability to train them using huge amounts of data is and has supercharged the field in recent years. Some of the bots trained on the roiling, messy mass of data that is the internet have, perhaps unsurprisingly, embarrassed their ‘parents’ by going on foul-mouthed tirades. And researchers have quickly discovered that while sophisticated chatbots such as ChatGPT could return sensible answers for basic scientific questions, they soon stumbled when challenged with something more technical. Instances such as these have led to LLMs being dismissed by critics as merely ‘plausible sentence generators’. So, training LLMs on the internet without filtering it for content and reliability clearly isn’t going to be a winner for creating a useful AI lab assistant. So what’s the alternative?
One obvious solution is to train them on trusted data sources. That’s what researchers have done with ChemCrow, an LLM that has learnt its trade using bona fide chemistry tools. Once connected up to an automated synthesis platform, ChemCrow could then be told to, for instance, make an insect repellent. It then conducted the research using trusted sources, planned a synthesis and carried it out to produce a known repellent compound.
LLMs are also being trained on chemistry journals too. In one case a ‘ChatGPT hunter’ was created that could tell whether a chemistry paper had been written using an LLM with impressive accuracy.
Despite examples such as these there’s still scepticism about LLMs usefulness in science. While ChemCrow’s demonstration was impressive, what it achieved wasn’t beyond the skills of a PhD candidate. And there are obvious concerns with outsourcing checks on manuscripts to a bot. More widely there’s the issue of reproducibility and AI’s black box nature – no one can say with certainty exactly what’s going on when these tools reach an answer. What’s needed is time to think. LLMs and their ilk are tools like any other. To use them properly will take time as we come to understand their limitations. Despite these cautionary words it’s hard not to feel a small frisson of excitement at the idea of an AI lab assistant. Bring on the future!
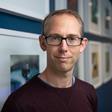
No comments yet