Artificial intelligence (AI) could help advance the computational prediction of chemical toxicity in aquatic organisms and eventually replace, or at least reduce, the use of animal testing in this area.
Chemical pollution is a major contributor to the worsening health of water systems across the world. However, determining the point at which a chemical’s concentration turns toxic to aquatic life requires intensive testing often involving animals.
‘We still know quite little about the toxicity of these chemicals; there is a huge knowledge gap,’ says Erik Kristiansson, a mathematician at the University of Gothenburg, in Sweden and one of the researchers on the study. ‘[But] to expand animal testing to all chemicals, especially when this number [is growing] exponentially, is not practically possible.’
Computational methods provide a fast and cost-efficient alternative but commonly used quantitative structure–activity relationship (QSAR) methods, that use regression or other predictive models to associate differences in chemical structures with changes in toxicological potency, are currently limited in their application.
To find a solution, researchers developed a ‘transformer’-based model that uses chemical structure, with effect measurements, to predict toxicity. Transformers are a deep learning methodology originally developed for natural language processing but, according to the researchers, they have been shown to be highly efficient at capturing information from biological and chemical structures.
The model was trained on a dataset consisting of 148,000 experimental measurements for 6500 chemical structures with species from three organism groups: algae, aquatic invertebrates and fish. On testing, the model was found to have high predictive performance of the toxicity threshold for each of the organism groups and, compared with three commonly used QSAR-based methods, was significantly more accurate and was able to make predictions for all structures in the evaluation dataset.
‘Using artificial intelligence, we can make much better predictions [about] whether a chemical is toxic or not for the environment, based on its structure,’ explains Kristiansson. ‘What we show in the study is that they already outcompete traditional QSAR-methods used to assess toxicity, and we strongly believe that this gap will continue to increase as more data is generated. We argue that we will reach a tipping point where these predictions are sufficiently accurate hopefully to then replace animal testing.’
‘The chemical economy is growing … [and] present level of chemical pollution have effects on human health and biodiversity,’ says Leo Posthuma, an expert in sustainability and environmental risks at Radboud University Nijmegen in the Netherlands. ‘[But] we lag behind in having methods available to evaluate them, alone or in combination; it is as if we test 10 car types, and declare 990 other types safe, or worse.
‘The use of artificial intelligence methods can be considered an important pathway to create a necessary breakthrough…. These methods, if well-designed and well-validated, can help describe the potential hazards of the chemical universe,’ Posthuma adds. ‘[It is] very important especially in the context of the EU-proposed concept of “safe and sustainable by design”.’
References
M Gustavsson et al, Sci. Adv., 2024, DOI: 10.1126/sciadv.adk6669
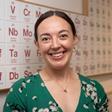
No comments yet