Artificial intelligence (AI) is transforming the pharma industry and its drug discovery pipeline
From the initial discovery to the final therapeutic, drug development is a notoriously expensive, time-consuming and difficult process. Current research estimates that just one in 5000 drug candidates make it through the drug discovery phase to licensing approval1, costing a projected $2.5 billion (£1.9 billion) per each new medication2. AI technologies are well positioned to streamline the complex pharma pipeline and provide cheaper routes to new drugs and innovative treatments. A growing number of pharma companies are turning to AI to enhance their drug discovery and development processes. However, given the importance of patents to the pharma industry, will AI-led advances in drug design create additional challenges for the intellectual property (IP) protection of the resulting innovations?
To navigate the multifaceted legal landscape, it is vital that pharma companies partner with experienced IP firms to ensure robust and well-developed patent strategies maximise potential revenue and valuation. Gill Jennings & Every LLP (GJE) – a leading IP firm based in London, UK and Munich, Germany – is dedicated to providing the pharma industry with the ideal combination of legal, technical and commercial expertise to help turn innovations into commercial success.
Artificial intelligence: real results
In order to fully understand the disruptive potential of AI, it is important to understand its background. AI is a branch of computer science that aims to build smart machines capable of performing tasks that require human intelligence. Since the 1960s, the life science sector has applied various AI technology approaches to discover new molecules for therapeutics. Today, supported by the rapid growth in computer-processing power, pharma researchers are beginning to see real results in the application of these methods.
AI systems can identify repurposing opportunities faster and more efficiently than traditional scientific research methods
To date, a major application of AI technologies in the pharma pipeline has been their use in repurposing existing drugs. This approach to developing novel medications involves examining new applications for therapeutics and drug substances that may already hold market and regulatory approvals for the treatment of a specific disease. Currently, identifying new therapeutic targets for drug products typically relies on a researcher’s expertise to understand whether a molecule may or may not interact with the target site. This requires biochemical expertise and is extremely labour intensive. Conversely, AI can rapidly run in silico experiments that test drug substances against multiple target sites and, based on clinical and patient data, highlight the potential new therapeutic applications that should warrant further human examination. When used in this way, AI systems help identify repurposing opportunities faster and more efficiently than traditional scientific research methods.
The use of AI in drug discovery poses important legal questions. If a novel therapeutic application is discovered through AI methodologies – and there’s enough data to support the filing of a patent application – who should be named as the inventor? Current patent law only recognises human ingenuity, and it does not view computers as sole creators. However, drug design is unlikely to be solely the result of an algorithm, and more conventional laboratory-based development will still be required. What is likely though, is that inventors may include scientists not only from the pharma company but also from the AI platform company.
Understanding the efficacy of drugs in new therapeutic areas provides greater scope for patent portfolio development
GJE sees great opportunities for innovation using AI tools. Having an increased understanding of the efficacy of drugs in new therapeutic areas provides greater scope for patent portfolio development, which strengthens the commercial position of pharma companies.
Sharing is caring
Another impactful application of AI technology in the pharma pipeline is drug property prediction. To accelerate the drug development process, early selection of ideal drug candidates is vital. Machine learning (ML) – a subset of AI technology – is the use of algorithms and statistical models to perform advanced tasks. By using these technologies in drug development, researchers can rapidly examine drug candidates based on multiple principles including potency, absorption, distribution, metabolism, excretion and toxicity (or Admet) properties and physicochemical characteristics.
For ML technologies to accurately predict drug properties, large and unbiased datasets are vital. This is because an algorithm trained purely on biased datasets that only contain positive results is inclined to think that all answers across the solution space would work. Unlike public domain datasets, pharma companies hold large amounts of unbiased data accumulated from clinical, biochemical and patient data. However, these large datasets are traditionally placed in rigid silos that are difficult to exploit. Therefore, a new approach to data collection – one which ensures it flows to the drug developers who most need it securely – is required.
This need for unbiased big data has encouraged pharma companies to take a novel and more collaborative approach to data handling. An example of this is the recent machine learning ledger orchestration for drug discovery (or Melloddy) project. The collaboration brings the insight, expertise and data of ten leading pharma companies, including AstraZeneca, Bayer and GlaxoSmithKline, together with seven major tech stakeholders. Drawing on this wide range of competencies, the consortium will develop a ML platform capable of leveraging multiple sets of proprietary data while respecting their highly confidential nature.
In any collaboration, determining the inventors can be a demanding task
As with any activity involving data, ownership and usage needs to be considered and the parties’ roles and responsibilities clearly defined. The growing collaborative nature between pharma companies and ML platform companies can create challenges when considering patent protection. This is because ownership of IP is designated initially by consideration of inventorship. In any collaboration, determining who the inventors are can be a demanding task. Overcoming these challenges will require the patent attorney to be more engaged in establishing inventorship and outlining partnership responsibilities.
Protecting patents
The AI-assisted revolution is beginning to transform how the pharma industry operates. With the expectation of greater innovation, the sector needs to work closely with patent attorneys to develop and implement robust IP strategies that leverage compound assets to maximise revenue streams.
At GJE, we work closely with both pharma companies and AI platform companies, helping them develop effective patent protection to support their drug design efforts.
John Jappy is a partner at GJE
As head of the chemistry and life sciences group, John helps companies of all sizes evaluate the business potential of their IP and works with them to secure patents relevant to their business aims. He works primarily in biotechnology and pharma and has advised on several IPOs and trade sales. He is considerably experienced in carrying out due diligence and freedom-to-operate analyses for companies and investors.
Ian Jones is an associate at GJE
Ian is a patent attorney and he handles a wide variety of work spanning the pharmaceutical and chemical fields but also extending into artificial intelligence, biotechnology and medical devices. A key aspect of this focuses on Patent Term Extensions and Supplementary Protection Certificates (SPCs) which extend market exclusivity for approved drugs in a range of jurisdictions worldwide.
References
1. Kraljevic, S., Stambrook, P. J., & Pavelic, K. (2004). Accelerating drug discovery. EMBO Reports, 5(9), 837–842. doi:10.1038/sj.embor.7400236
2. J DiMasi et al (2016). Innovation in the pharma industry: New estimates of R&D costs. Journal of Health Economics, 47, 20–33. doi:10.1016/j.jhealeco.2016.01.012
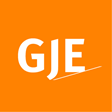
1 Reader's comment