A new AI model, React-OT, could revolutionise computational chemistry by predicting transition states with remarkable accuracy, all while completing the task in under a second and using far less computational power than conventional methods.
‘Think of a chemical reaction as hiking across a landscape of mountains and valleys,’ explains corresponding author Yuanqi Du of Cornell University. ‘The transition state is a saddle point – the lowest point along a high mountain ridge that connects two valleys. Finding it is hard because it’s a very unstable spot: take even a small step in one direction and you’ll fall down into one valley.’
Transition states are notoriously elusive, existing for just femto- or picoseconds before vanishing. ‘Only ultrafast spectroscopy can capture a few examples,’ says Chenru Duan at the Massachusetts Institute of Technology, another corresponding author. Yet understanding transition states is crucial as they define the energy barrier between reactants and products, shaping both the mechanism and speed of a reaction.
Traditionally, chemists have predicted transition states by calculating the minimum energy pathway – a kind of energetic bridge between reactants and products. This involves tracing the most likely route a chemical system will take from start to finish. When scientists only know the structures of the starting molecules and products, they use computational methods to estimate the reaction path. While these methods are valuable, ‘these calculations typically require thousands of energy and force evaluations, making them highly time-consuming’, says Du.
A new approach
To tackle the problem, Du, Duan and colleagues developed a new computational method that uses a type of generative AI known as a diffusion model. These models work by adding noise to training data and then learning how to reverse the process, effectively reconstructing the original input step by step.
‘Instead of using Gaussian noise as the initial guess for transition states, React-OT employs linear interpolation of reactants and products, akin to the educated initial guesses used in classical optimisation methods,’ explains Guan-Horng Liu at the Georgia Institute of Technology, also a corresponding author. ‘We then train a flow model that smoothly and deterministically transports samples from these educated guesses to the transition states.’
React-OT was tested on a publicly available dataset containing just over 10,000 diverse organic reactions and pairs of reactants, transition states and products. ‘No surprise, we got state-of-the-art results,’ says Duan. ‘But we did not stop there. We pushed React-OT to study a real-world reaction network problem: the decomposition of γ-ketohydroperoxide.’
The team found React-OT was not only highly accurate, but incredibly fast. ‘The improvements seem impressive,’ comments Timothy Jenkins at the Technical University of Denmark who was not involved in the study. ‘Most existing methods … use quantum chemistry, which is accurate but very slow. Where other methods might take minutes to hours per molecule, React-OT does it in under a second.’
React-OT builds on an earlier model, OA-ReactDiff, created by Duan in 2023. ‘OA-ReactDiff achieves impressive performance in predicting [an] accurate transition state,’ he says. ‘However, it carries the stochastic character of diffusion models, meaning that we do not get a deterministic answer. This drove us mad because chemistry is precise science.’
Taking prediction to the next level
The breakthrough came when Duan encountered a study by Guan, who was using a mathematical technique called optimal transport in image generation, and realised this was exactly what he needed to take OA-ReactDiff to the next level. ‘Instead of transforming Gaussian noise to target distribution, we need to start with an educated guess from chemistry, then do optimal transport to the target,’ Duan explains. The result was React-OT – an AI model up to 1000 times faster at identifying transition states, without sacrificing accuracy. ‘A straight comparison for single transition state structure generation would conclude that React-OT is 60% more accurate with a 20-fold speedup,’ adds Duan.
‘These are important improvements, especially when this type of method is used to solve real-world problems, like multi-step chemical reactions or even protein folding,’ comments Kari Laasonen at Aalto University, who was not involved in the study. ‘The training dataset is much larger than in previous studies, and this is important for good predictability. It will be very interesting to see how React-OT will perform in complex chemical applications.’
‘Right now, it solves a focused problem,’ adds Jenkins. ‘But the approach is general enough to expand. The principles behind React-OT could eventually find use in modelling conformational changes in biomolecules or in designing catalytic proteins that guide reactions through specific transition states.’
The researchers see generative AI as a powerful tool for reimagining chemical discovery – shifting from traditional screening to actively exploring and programming chemical reactions. ‘Generative AI provides [a new way of thinking] about how chemistry can be done,’ concludes Duan. ‘Instead of just screening the vast space of chemical reactions, we can now truly explore.’
References
C Duan et al, Nat. Mach. Intell., 2025, 7, 615 (DOI: 10.1038/s42256-025-01010-0)
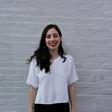
No comments yet