Researchers in China have developed a machine learning tool that can predict both the stereoselectivity and activity of engineered enzymes by analysing their amino acid sequence. They claim their tool can dramatically reduce the experimental workload of scientists using directed evolution to develop biocatalysts.
Enzymes are increasingly being used to catalyse the production of chemicals that have previously been derived via traditional synthetic processes, thanks in part to their ability to work in water. But natural selection only produces enzymes that perform as well as they need to for their natural purpose. This means that naturally-occurring enzymes rarely perform as well as industrial catalysts. That’s why scientists often engineer enzymes to have more favourable properties, such as better selectivity, higher turnover numbers or even completely new catalytic functions.
The most common way of improving enzymes for industrial applications is directed evolution, which effectively mimics natural selection in a laboratory. But while this method is popular and effective, it is inefficient in terms of time or resources. Here computational tools can help.
A team around Chun-Yue Weng and colleagues at Zhejiang University of Technology has developed a computational framework – called UniESA – that can predict an enzyme’s stereoselectivity and activity from its amino acid sequence. The team describe UniESA as a universal framework capable of predicting functions across various enzymes and that this universality makes it stand out from previous tools. ‘UniESA demonstrated improved performance in all the evaluation metrics in two tested datasets, compared to its predecessor,’ states Wang.
However, Carlos Acevedo-Rocha, a senior researcher in protein engineering at the Technical University of Denmark, disputes the claims by the UniESA researchers that the system achieved universality. The UniESA researchers claim universality because they tested in silico a number of different enzyme systems already described in the literature, but Acevedo-Rocha points out that in each case, UniESA is trained using experimental data that includes the stereoselectivity of known mutants of the enzyme being predicted. Therefore, when the predictions are subsequently made by UniESA, it is unsurprising that they have a good correlation with the dataset. To achieve true universality, the system would need to predict the stereoselectivity of mutants of other enzyme systems not tested in the lab previously. ‘UniESA is not making a significant advancement because it is only predicting mutations that are very close to the ones previously found in the same enzyme system,’ says Acevedo-Rocha. He also points out that other tools have been used to predict enzyme stereoselectivity, including the Modify system, developed last year by researchers in the US.
So will computational models eventually replace directed evolution? Weng thinks not. While computational models are able to predict the properties of enzymes, lab-based experiments are still needed for validation and to provide data. ‘Especially when the data for a specific enzyme is quite limited, the lab-based directed evolution remains as an important and indispensable approach,’ says Wang.
Acevedo-Rocha on the other hand, says that it will: ‘In a not-so-distant future, we will be able to design efficient enzymes using computer-based methods.’
References
C-Y Weng et al, Green Chem., 2025, 27, 3777 (DOI: 10.1039/d4gc06253a)
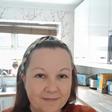
No comments yet