The brain is often regarded as a soft-matter chemical computer, but the way it processes information is very different to that of conventional silicon circuits. Three groups now describe chemical systems capable of storing information in a manner that resembles the way that neurons communicate with one another at synaptic junctions. Such ‘neuromorphic’ devices could provide very-low-power computation and act as interfaces between conventional electronics and ‘wet’ chemical systems, potentially including neurons and other living cells themselves.
At a synapse, the electrical pulse or action potential that travels along a neuron triggers the release of neurotransmitter molecules that bridge the junction to the next neuron, altering the state of the second neuron by making it more or less likely to fire its own action potential. If one neuron repeatedly influences another, the connection between them may become strengthened. This is how information is thought to become imprinted as a memory, a process called Hebbian learning. The ability of synapses to adjust their connectivity in response to input signals is called plasticity, and in neural networks it typically happens on two timescales. Short-term plasticity (STP) creates connectivity patterns that fade quite fast and are used to filter and process sensory signals, while long-term plasticity (LTP, also called long-term potentiation) imprints more long-lived memories. Both biological processes are still imperfectly understood.
Neuromorphic circuits that display such learning behaviour have been developed previously using solid-state electronic devices called memristors, two-terminal devices in which the relationship between the current that passes through and the voltage applied depends on the charge that passed through previously. Memristors may retain this memory even when no power is applied – they are ‘non-volatile’ – meaning that neuromorphic circuits can potentially process information with very low power consumption, a feature crucial to the way our brains can function without overheating. Typically, memristor behaviour manifests as a current–voltage relationship on a loop, and the response varies depending on whether the voltage is increasing or decreasing: a property called hysteresis, which itself represents a kind of memory as the device behaviour is contingent on its history.
Fluid thought
Lydéric Bocquet of the Ecole Normale Supérieure in Paris and co-workers,1 and independently Ping Yu of the Chinese Academy of Sciences’s Institute of Chemistry in Beijing and colleagues,2 have now made memristors that work using aqueous solutions of ions. They are not the first to do this,3,4 but the new devices work with low voltages and show versatile memory effects that truly mimic the plasticity and learning functions of neurons.
In the devices of Bocquet and colleagues, the solution flows through slit-like nanochannels made from atomically smooth flakes of molybdenum disulfide or activated carbon just a few nanometres wide, to the walls of which a voltage can be applied. The flakes sit on a silicon nitride membrane pierced by a microscopic pore, through which a salt solution passes. The ion current through the slit depends on the applied voltage in a highly nonlinear manner with a hysteresis loop, thus offering memristor behaviour – a possibility that Bocquet and his co-workers had shown previously in principle, but without making working devices.5
The researchers attribute this behaviour either to interactions between ions in the confined space, or to an asymmetry in the ways ions pass in each direction, depending on the type of device used. If a voltage ‘write’ pulse lasting just 10 seconds is applied to the activated-carbon device, its ion conductance initially increases before rapidly decaying in less than two minutes. It doesn’t decay back to its original level but reaches a higher plateau where it might stay for several hours. Thus there is both short-term and long-term plasticity in the device in response to a kind of artificial action potential. Bocquet and colleagues used this behaviour to demonstrate Hebbian learning, where the conductance only changed if two sets of pulses arriving at the two terminals of the device were correlated in the right sequence, mimicking the learning that happens if two neurons at a synapse ‘wire together when they fire together’.
In the nanofluidic devices of Yu and co-workers, the ionic solution passed through the circular mouth of a nanopipette, to the walls of which were attached chains of the polymer polyimidazole. These positively charged chains can bind the negative ions in solution, and the dynamics of adsorption again create a hysteretic response that can be used to store short-term memories. ‘The short-term plasticity originated from the history-dependent ion distribution in our device,’ says Yu. Because the adsorption here depends on the chemical interactions of the ions and the tethered polymers, the memory characteristics can be changed by tuning the chemistry of the system. In one example, the researchers showed that the memory effect persists in an ionic solution comparable to physiological fluids, but is changed by adding the ‘energy biomolecule’ adenosine triphosphate (ATP). This illustrates an ability to interface and ‘speak’ to biological systems. They also showed how a chemical signal – a change in the concentration of an anion – could be converted into an electrical response, mimicking the activation of a neuron by neurotransmitters at a synapse.
Storage capacity
Such biocompatibility is perhaps even more evident in the neuromorphic memory effects described by Patrick Collier of Oak Ridge National Laboratory in Tennessee and colleagues.7 Building on their earlier work,3,6 the researchers have now shown both short-term6 and long-term7 plasticity in systems of two droplets of an aqueous salt solution, a few hundred micrometres across, coated with a layer of lipid surfactants and suspended in an organic solvent. When brought into contact, the droplets stick together to form a lipid bilayer at the interface, like that of cell membranes. They found that the capacitance across the membrane also exhibits hysteresis and plasticity, because of rearrangements of the lipid molecules and ions in the bilayer. These circuits thus act not so much as memristors, but memcapacitors: the charge that they store and release is dependent on the past in a way that mimics short-term plasticity. Given that the dry weight of brain tissue is around 50% lipid membrane, the researchers think it is not inconceivable that such memory effects might even play a role in biological neural networks.
‘Both short- and long-term plasticity are important for giving neural networks different levels of computation and memory,’ says Timothée Lévi, a specialist in neuromorphic computation at the University of Bordeaux. He says that, while chemical systems like these operate more slowly than solid-state devices, such as silicon, they can potentially operate at lower power levels. ‘Fluidic devices can be very efficient and scalable, and could be the future of neuromorphic systems,’ he says.
After all, says Bocquet, the brain works with the energetic equivalent of two bananas a day: far less than conventional computers. But biophysicist Joseph Najem of Pennsylvania State University cautions that that current memristor devices ‘don’t have any advantage over silicon circuits when it comes to power consumption – while they operate at low voltages [around 2V] and generate low currents [nanoamps], their power consumption would not be too far from that of silicon’. Their real advantage, Najem says, might be in interfacing with biological systems.
‘The fact that the brain is using ions and water to make its calculations, instead of electrons, is quite remarkable,’ says Bocquet. He believes that the new devices offer ‘an opportunity to dig into ionic computing and really investigate what its performance is’. The next step, he adds, is to create networks of these devices – or to connect them with real neurons. Yu has the same goal. ‘We hope to use these devices not only to read and process chemical information from neurons, but also to deliver stimulation to real neurons to control them,’ he says.
References
1 P Robin et al, Science, 2023, 379, 161 (DOI: 10.1126/science.adc9931)
2 T Xiong et al, Science, 2023, 379, 156 (DOI: 10.1126/science.adc9150)
3 JS Najem et al, ACS Nano, 2018, 12, 4702 (DOI: 10.1021/acsnano.8b01282)
4 P Zhang et al, Nano Lett., 2019, 19, 4279 (DOI: 10.1021/acs.nanolett.9b00525)
5 P Robin, N Kavokine and L Bocquet, Science, 2021, 373, 687 (DOI: 10.1126/science.abf7923
6 JS Najem et al, Nat. Commun., 2019, 10, 3239 (DOI: 10.1038/s41467-019-11223-8)
7 HL Scott et al, Proc. Natl Acad. Sci. USA, 2022, 119, e2212195119 (DOI: 10.1073/pnas.2212195119)
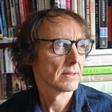
No comments yet