A machine learning model has been created that can identify ruthenium-based antibiotic drug candidates. With a small training set of just 288 antibacterial organometallic compounds, the algorithm scanned millions of structures, selecting the most active against resistant bacteria. The most promising candidates were tested and showcased almost six times greater antibiotic activity than the training set.
Antibiotics have become ‘a cornerstone of most modern medicine, as many hospital treatments rely on antibiotics as a measure to control infection’, says lead author Angelo Frei from the University of Bern in Switzerland. However, growing bacterial resistance to these drugs has become a serious problem. Recently, researchers have recognised the potential of metal-based antimicrobials – including ruthenium complexes. Compared with traditional organic carbon-based chemicals, metal compounds are 10 times more likely to be active against bacteria and are not necessarily more toxic to humans, explains Frei. ‘They represent a vast compound class that has remained largely unexplored for its use in medicine,’ he adds. Ruthenium compounds are also simple to synthesise, making them easier drug candidates to explore.
Frei says that the team first used a combinatorial chemistry approach developed by co-author Wee Han Ang to create a library of 288 ruthenium compounds, which were then tested against methicillin-resistant Staphylococcus aureus (MRSA). ‘We found a substantial amount to be active (9.4%), and … used this data to train machine-learning models to predict the activity against MRSA,’ he adds. After these first steps, researchers built a virtual library of 77 million ruthenium complexes. The algorithm then identified two million potentially active structures. To verify the predictions, the team assembled a smaller sample of 54 structures and tested them in the lab against MRSA. ‘53.7% of these compounds were active, which represents a 5.7x higher hit rate than the initial screening,’ comments Frei.
‘Organometallic compounds often have distinct mechanisms of action compared to traditional organic antibiotics, which could be advantageous to … overcome existing resistance mechanisms,’ explains Concepción Gimeno, an expert in metallodrugs at the Institute of Chemical Synthesis and Homogeneous Catalysis in Zaragoza, Spain. Ruthenium complexes’ interesting properties include ‘biocompatibility and a very low toxicity compared to other metal complexes’, adds Gimeno. Ruthenium complexes are already being investigated in clinical trials for cancer.
Nils Metzler-Nolte, an expert in bioinorganic chemistry at Ruhr University Bochum, Germany, admires the versatility of the method. ‘Building upon previous work in combinatorial chemistry by the Ang group … a simple one-pot reaction gives over 250 compounds with vastly different 3D shapes and properties,’ he explains. ‘This is quite unmatched when you consider the three-dimensional space mapped out with these compounds.’ This is an attractive aspect of organometallic complexes – ‘compounds with radically new structures and chemical properties [could offer] antibiotics with new and unprecedented modes of action’, says Metzler-Nolte.
Although ruthenium is relatively expensive and scarce, the syntheses are only between one and three steps, which is very economical compared with commercially available drugs. ‘Moreover, the cost of drug discovery and development is not dictated by the cost of the synthesis, but rather by the huge cost of clinical trials,’ Metzler-Nolte points out.
Follow-up studies ‘will involve a series of experimental and computational validations to confirm and refine the predictions, then synthesis, characterisation, biological tests, iterative design and more,’ says Gimeno. Perhaps most importantly, molecular simulations will help understand the unusual antibiotic mode of action of these metal complexes, as well as any resistance observed. ‘Some studies show a very low – even non-existent – development of resistance for metal-based compounds, but I think it would be foolish to underestimate bacteria,’ says Frei. ‘Our first aim is to generate more data and larger libraries … to cover more of the periodic table and … predict more specific properties, such as the degree of activity and toxicity.’
References
M Orsi et al, Angew. Chem. Int. Ed., 2023, DOI: 10.1002/anie.202317901
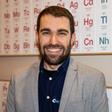
No comments yet