Algorithm learns chemistry just like a human and can predict the outcome of reactions it has never seen before
A new neural network – a kind of artificial brain – can predict the outcome of organic reactions. Although this new algorithm may not be the most accurate around, it is certainly the most flexible: it learns organic chemistry the way humans do.
What’s more, scientists don’t need to tweak the algorithm to expand its understanding of chemistry. Instead, they just need to ‘teach’ it a lot of chemical reactions to give it a good grounding in the field. Then, the neural network can solve problems it has never encountered before. It can identify the important functional groups in the reactants, compare them with what it previously learnt and finally predict the outcome of the reaction.
Our algorithm is like a kid learning how to eat spaghetti. It’s still dropping some
Alán Aspuru-Guzik, Harvard University
To test this new system, researchers taught the algorithm two important units in any organic chemistry course: reactivity of alkenes and alkyl halides. Then, just like any other chemistry student, the program had to take a test. The authors selected two problems out of the popular organic chemistry textbook simply known to most students as Wade’s. The algorithm passed the exam with flying colours, answering 80% of the questions correctly.
The team, led by Alán Aspuru-Guzik at Harvard University in the US, wants to bring machine learning, a technique that is already being used in voice recognition and data-mining, to bear on organic chemistry. ‘Using machine learning we hope not to need any templates,’ says Jennifer Wei, first author of the paper. ‘In the future, our algorithm should be able to recognise any reaction and extrapolate the outcome from the pathways it already knows.’
‘Neural networks will soon be creative, and may give you answers that you didn’t teach them in the first place,’ comments Aspuru-Guzik. ‘For example, we didn’t teach our program the reactivity of cyclic hydrocarbons, and yet it was able to come up with some answers for this kind of reactions.’ Aspuru-Guzik notes that this promising field is still in its infancy and says ‘our algorithm is like a kid learning how to eat spaghetti. It’s still dropping some, but after seeing how it’s done time after time, it will eventually learn how to do it.’
Bartosz Grzybowski, distinguished professor at UNIST in Korea and creator of chemistry brain Chematica, finds this new paper ‘a very interesting approach, although the algorithm may still need further training in learning new reactions to become really accurate.’ Grzybowski says there’s still work to be done and ‘the program should learn to pick up on the subtle differences in molecules that sometimes trigger a butterfly effect leading to totally unexpected products.’
Aspuru-Guzik knows the limitations of their approach but he wants to keep developing understudied areas of theoretical chemistry. ‘We want to explore the power of neural networks in chemistry,’ he says. ‘Chemical reactions are not our only project, we are also using machine learning to design new materials, and very recently we published our latest results on how to use neural networks to design new drugs.’ Aspuru-Guzik says that, while it’s still early days, his team has high hopes for its neural net: ‘Although it may take us five to 10 more years, our long-term goal would be to beat any other synthetic prediction system.’
References
J N Wei, D Duvenaud and A Aspuru-Guzik. ACS Cent. Sci., 2016, DOI: 10.1021/acscentsci.6b00219
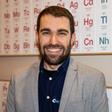
No comments yet