A deep learning neural network trained on 50,000 crystal structures of inorganic materials has acquired the ability to recognise chemical similarities and predict new materials.
One way to find out whether two elements from the periodic table will form a crystalline material is the tried and trusted ‘shake and bake’ – mix them together at a range of different stoichiometries and hope for the best. Binary materials are thus very well covered in the scientific literature, but this method can’t keep up with the vastly more complex combinatorial possibilities afforded by three or more elements.
Therefore, predictions of which elements will combine in which ratios to form regular solids are necessary and they hold the promise of new materials with desirable or even unprecedented properties. Current prediction methods typically use evolutionary algorithms and apply them to random starting structures. These approaches rely on lengthy energy calculations, which makes them difficult to deploy quickly.
Kevin Ryan, Jeff Lengyel and Michael Shatruk at Florida State University, US, have developed a novel approach to this problem using a deep neural network. This complex type of artificial intelligence is responsible for the recent resurgence of interest in machine learning and the emergence of self-driving cars.
They trained the network on 50,000 inorganic crystal structures without giving it any knowledge of chemical theory, leaving it to figure out the chemistry from the geometrical arrangements of atoms in crystals alone. Tests revealed that the network learned to recognise the similarities within the groups of elements in the periodic table.
‘Neural networks, in general, develop their own internal, data-driven representation during training,’ explains Ryan, a graduate student who developed the model. ‘In the present case, the network developed its own representation from a new kind of fingerprint which conveyed the three-dimensional information about an atomic site’s environment. The new fingerprint can be thought of as 12 virtual ‘eyes’ surrounding the site to give the network multiple perspectives.’
The network uses the chemical knowledge it gained during training to identify which hypothetical, combinatorially generated crystal structures are the most reasonable. The network’s success was judged by how well it could identify examples of real crystals it had never seen during training from large sets of decoys. The researchers found that in 30% of the cases it ranked at least one known compound among the 10 most likely possibilities. In contrast to other structure prediction methods, the model’s performance was not limited to small, hand-selected subsets of structures.
‘The resulting prediction model is appealing due to its nearly real-time evaluation that can be carried out on affordable personal computers. To perform a prediction, the user simply enters a desired set of chemical elements, and the program returns a list of results in seconds,’ Ryan explains. This list provides a manageable set of suggestions among the astronomical number of possible combinations of three or more elements. These may not be correct and definitive answers, but researchers can readily test them experimentally, and they may lead to the discovery of new materials with interesting properties.
Artem R Oganov from the Skolkovo Institute of Science and Technology in Russia, who in his earlier work developed the structural fingerprints used in this study, welcomed the advance cautiously. ‘It’s a good paper, but we’re still at the beginning,’ he says. The main problems with it, according to Oganov, are the fact that the representations are not necessarily unique to a specific structure and the relative incompleteness of the existing data when compared with the possible combinations of elements. ‘There are still important problems to be solved before it can become everybody’s tool.’
References
K Ryan, J Lengyel and M Shatruk, J. Am. Chem. Soc., 2018, DOI: 10.1021/jacs.8b03913
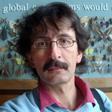
No comments yet