Computers and chemists have been friendly for a long time. But when it comes to drug discovery, current computational methods are often too drawn out and inefficient to identify the mechanism of action of drugs. Now, a multidisciplinary team of researchers believes artificial intelligence could help out in pharmacology studies. The team’s new approach successfully identified a potent inhibitor for 5-lipoxygenase, an enzyme that is over-expressed in a range of human tumours.
Identifying how drug candidates interact with their target to treat disease is key to drug development. ‘[This] is a bottleneck in drug discovery and it is typically done through proteomic approaches, which tend to be very laborious, time consuming and do not guarantee success in regards to linking drug target binding to modulation of disease,’ explains Gonçalo Bernardes, a researcher at the University of Cambridge, UK, and one of the lead authors of the study. He explains that artificial intelligence ‘also provides research hypotheses that need to be experimentally confirmed, but in a much faster and economical way’.
Co-author Tiago Rodrigues, at the University of Lisbon, Portugal, says that machine learning can go way beyond more classical molecular docking. ‘[Our] tool does not depend on the structure of proteins, so it can be applied in cases where molecular docking might not,’ he says. This new artificial intelligence tool is also quicker, hence cheaper. ‘Docking tends to be computationally expensive, whereas we can profile one molecule against thousands of drug targets in less than 10 minutes,’ he adds.
Bernardes and his team relied on a huge database of compounds and drug targets they used to ‘teach’ a single desktop computer. Researchers used two different machine learning methods: ‘One gives a bind/don’t bind answer … and the other uses several decision trees to predict an affinity value,’ explains Bernardes. Then, the algorithm gives a prediction. In this case, it suggested likely targets for the natural product β-lapachone – among them enzyme 5-lipoxygenase. ‘Machine learning allows us to leverage statistical patterns found in data,’ explains Alán Aspuru-Guzik, an artificial intelligence researcher at the University of Toronto and co-founder of Zapata Computing, a start-up specialising in quantum computing algorithms. ‘When high-quality datasets exist, machine learning can model these phenomena much faster and cheaper … accelerating efforts for the discovery of novel drugs,’ he explains.
The team’s chemists also synthesised a set of eight β-lapachone analogues, and tested their binding affinity to 5-lipoxygenase. None of them outperformed β-lapachone anticancer activity, however. The algorithm had found a perfect match. This highlights the importance of the structure and substitution pattern for bioactivity. To further analyse how β-lapachone binds to its target enzyme, Bernardes and co-workers created enzyme models and carried out computational studies that confirmed what they had found in the lab – β-lapachone binds strongly to the enzyme’s active site.
Benjamin Sánchez-Lengeling, a PhD candidate in Aspuru-Guzik’s lab, says that in future ‘artificial intelligence will become essential in the search for, and development of, new ligands and drug candidates’. Chemistry labs will almost certainly change thanks to these new techniques. ‘Artificial intelligence will be integrated into all aspects: simulation, experimental planning and characterisation,’ adds Sánchez-Lengeling. Bernardes agrees: ‘We have applied [our artificial intelligence approach] to four other projects, and one of them is fairly advanced.’
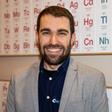
No comments yet